We will be part of the FAIR PNRR project in Spoke 5
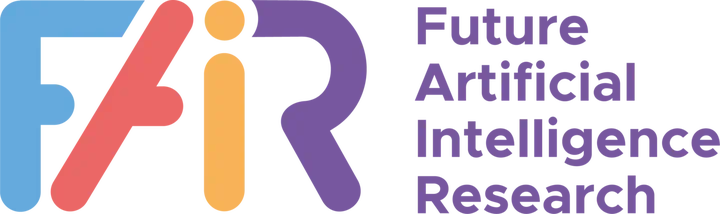
We will be part of the FAIR (Future of AI research) PNRR project in Spoke 5
FAIR: Future of AI Research - SPOKE 5: High Quality AI
The “Future Artificial Intelligence Research (FAIR)” project aims to contribute to addressing the research questions, methodologies, models, technologies and also the ethical and legal rules to build Artificial Intelligence systems capable of interacting and collaborate with humans, to perceive and act within constantly evolving contexts, to be aware of one’s limits and capable of adapting to new situations, to be aware of safety and trust perimeters, and to be attentive to environmental impact and social that their creation and execution may entail.
Robust and semantic-aware representation learning
Learning-based Artificial Intelligent (AI) systems have made extraordinary leaps in solving highly complex cognitive tasks with superhuman performance, such as correctly recognizing a face image among millions or generating synthetic images that humans find difficult to distinguish from natural images. However, several open problems still need to be solved in the future relating to the impact of AI on society and the quality of AI. These problems include the robustness of those systems, how to preserve human privacy given the recent misuse of AI, and how to make AI explainable and reusable. We attack all these problems using the lens of adversarial machine learning and plan to use robustness as the leitmotif of the research.
Goal:
The goal of this task is to analyze, study and experiment with the robustness of a diverse set of deep learning models applied to different domains: Euclidean domain, e.g., images, domains where the data naturally form a sequence, and even non-Euclidean domain (graphs and manifolds). We do so by emphasizing the robustness of a model and its capability of learning the density of the input data, thereby studying the connection between robust models (i.e., models resilient to adversarial attacks) and generative models. We expected to apply the studies to supervised and self-supervised learning scenarios.
Directions and expected achievements:
We plan research work along the following directions: i) study the relationship between robust, discriminative models and generative models in the form of Energy-Based Models (EBM), analyzing the relationship between adversarial attacks and score matching ii) develop novel attacks that exploit the notion of data density and deform the input with non-semantic bound (classic L_p norm attack) and semantic bound (e.g., attacks that perturb the data over latent, meaningful attributes). We also plan to explore non-contrastive techniques as an alternative to adversarial training. Results are expected to reduce the gap between discriminative and generative models and make AI more robust yet effective.
Keywords: robust models, adversarial attacks, 3D, representation learning